Statistics 101 - The Quick Path to Statistics for 2023
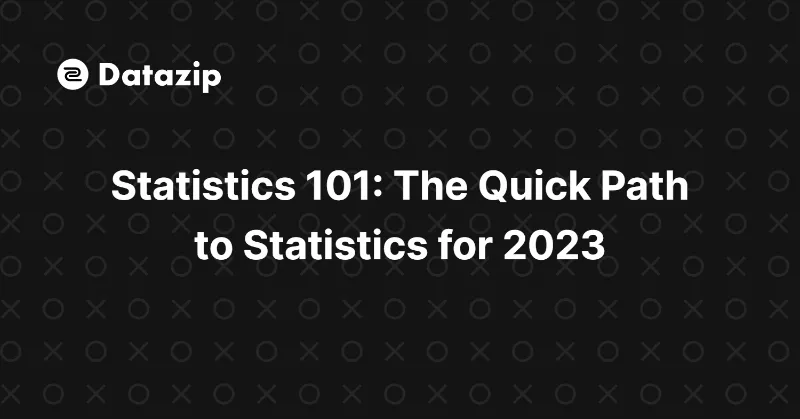
Check out OLake, our latest open source offering for replicating databases to lakehouses.
Statistics!! Math again!! Hey hey, I promise this will not freeze your brain but rather give you a clear path to statistics. So without further ado, let's dive in. This article will mainly focus on useful statistical terms in the marketing world.
What does Statistics mean?
Statistics is a branch of mathematics that deals with data collection, analysis, interpretation, presentation, and organization. It is used in various fields, including science, engineering, finance, and economics, to make informed decisions and predictions based on data.
Some key statistical concepts include probability, random sampling, hypothesis testing, regression analysis, and statistical inference.
These techniques help researchers and analysts understand complex data sets and draw conclusions supported by the available evidence.
Use of Statistics in Marketing
Marketing makes use of statistics to determine market trends as well as to gauge the effectiveness and potential of marketing initiatives.
Accurately identifying the target market and using efficient communication channels and methods to reach them is crucial to good marketing.
The marketer can use statistics to help them accomplish both of these objectives, as well as to assess the effectiveness of their marketing strategy and to offer information on which to base future modifications to their current marketing plan.
A marketing strategy is a long-term plan for achieving a company's objectives by comprehending customer needs and forging an identifiable and long-lasting competitive advantage.
This includes everything from deciding who the target audience is to select the channels one will use to communicate with them.
With the help of a marketing strategy, one can specify how the business will position itself in the market, the kinds of products they will make, the strategic alliances they will forge, and the forms of advertising and promotion they will use.
Any business that wants to succeed must have a marketing strategy.
Statistics plays a vital role in marketing, as it allows marketers to make data-driven decisions and understand the effectiveness of their marketing campaigns. Some specific ways in which statistics are used in marketing include:
- Measuring the success of marketing campaigns by collecting and analyzing data on metrics such as clicks, conversions, and sales.
- Identifying patterns and trends in customer behavior, such as buying habits and preferences, by analyzing customer surveys, focus groups, and market research studies.
- Developing predictive models to forecast future market conditions and customer behavior based on data from past marketing campaigns and other sources.
- Evaluating the effectiveness of different marketing strategies and tactics, such as advertising, promotions, and email marketing, by analyzing how customers respond to these initiatives.
- Developing segmentation strategies to target specific customer groups based on data on their characteristics and behavior.
Overall, statistics is an essential tool for marketers, as it allows them to make data-driven decisions and better understand their customers and the market.
Types of Statistics
There are many different types of statistics, and the type of statistics used in a given situation will depend on the data being analyzed and the research question being addressed. However, some common types of statistics include:
- Descriptive statistics summarize and describe the characteristics of a data set, such as the mean, median, and mode.
- Inferential statistics use sample data to make inferences about a population, such as estimating a population means or testing a hypothesis about a population parameter.
- Regression analysis models the relationship between two or more variables, such as predicting the likelihood of an event occurring based on certain factors.
- Time series analysis examines data collected over time, such as analyzing trends or forecasting future values.
- The multivariate analysis examines the relationships between multiple variables, such as identifying factors influencing customer behavior.
Overall, there are many different types of statistics, and the appropriate type of statistics to use in a given situation will depend on the data being analyzed and the research question being addressed.
One cannot master statistics without knowing its ABCs. Let us now read in depth about the various terms used in statistics.
Measures of Central Tendency
First, we'll deal with simple descriptive statistics confined to one variable. Let us start with measures of central tendency, i.e., the Mean, Median, and Mode.
The mean is the average of the given numbers and is calculated by dividing the sum of the given numbers by the total number.

The median is the middle value of the given list of data when arranged in an ordered list. The arrangement of data or observations can be made either in ascending or descending order.

The modal is the value or number in a data set that has the highest frequency or appears most frequently.
- The set is called bimodal when there are two modes in a data set**.**
- The set is called trimodal when there are three modes in a data set**.**
- The set is called multimodal when there are four or more modes in a data set**.**
Measures of Dispersion
Measures of central tendency alone do not adequately describe the variable. The other dimension of a variable is its dispersion or spread. There are three measures of dispersion: Range, Variance, and Standard Deviation.
The range can be defined as the difference between the highest and lowest observations. The obtained result is called the range of observation. The range in statistics represents the spread of observations.

The variance is a measure of how data points differ from the mean. It calculates how far apart data points are from their mean value.
The variance is the square of the standard deviation, i.e.,

The corresponding formulas are hence,
- Population standard deviation:

- Sample standard deviation
The standard deviation is a measure that shows how much variation there is from the mean.

Relationship Between two Variables: Covariance and Correlation
Looking at the word "relationship," many will feel butterflies in their stomach. For a relationship to work, both parties need to be stagnant; that is what we have seen so far. Now let's see how the relationship between two variables will look.
Covariance
Covariance measures the relationship between two random variables and to what extent they change together. Covariance can have both positive and negative values. Based on this, there are two types:
- Positive Covariance: If the covariance for either of the two variables is positive, both variables move in the same direction. That is, if the values of one variable (greater or lesser) correspond to the values of another variable, they are said to be in positive covariance.
- Negative Covariance: If the covariance for any two variables is negative, both variables move in the opposite direction. It is the opposite case of positive covariance, where greater values of one variable correspond to lesser values of another variable and vice versa.

Correlation
A correlation is a statistical measure that identifies the strength and direction of a relationship between two or more variables.
However, just because two variables are correlated does not necessarily imply that the change in one of the variables changed the values of the other. There can be three situations to see the relation between two variables -
- Positive Correlation - when an increase or decrease in the value of one variable is followed by an increase or decrease in the value of the other variable.
- Negative Correlation - when the values of the two variables move in opposite directions so that an increase or decrease in one variable is followed by an increase or decrease in the other.
- No Correlation - when there is no linear dependence or no relation between the two variables.

Learn how Datazip is transforming customers' lives.
Probability and sampling distribution
Probability means possibility. It is a measure of the likelihood of an event occurring. The probability of all the events in a sample space adds up to 1.
- Probability of event to happen P(E) = Number of favorable outcomes/Total number of outcomes
A sampling distribution is the probability distribution of a given random-sample-based statistic. There are three types of sampling distribution -
- Sampling Distribution of Mean - The average of every sample is put together, and a sampling distribution mean is calculated, reflecting the nature of the whole population.
- Sampling Distribution of Proportion - The mean of all sample proportions is calculated, thereby generating the sampling distribution of proportion.
- T-Distribution - Under this type of sampling distribution, the population size is very small, leading to a normal distribution.
Regression
Regression is a statistical method that helps us analyze and understand the relationship between two or more variables of interest. Logistic regression is a statistical model used to predict an outcome's probability.
Regression in general and logistic regression have several characteristics. For instance, they are both single equations and have diagnostics regarding the effects of independent factors on the dependent variable and 'fit' diagnostics.
They also have both dependent and independent variables and are single equations.
But there are a lot of other ways they differ. The dependent variable in logistic regression can only take on the binary values of 0 or 1.
When calculating the coefficients for logistic regression, the maximum likelihood method using a grid search is used rather than the standard criteria of "minimizing the sum of the squared errors."
Finally, there are differences in how the coefficients are interpreted. Odds ratios are frequently used, and the fit is not based on comparing a predicted dependent variable to one that exists.
Issues with Regression Modeling
Several potential issues can arise when using regression modeling, including:
Nonlinear Relationships
Regression models assume that the relationship between the dependent and independent variables is linear. However, the relationship may not be linear, leading to inaccurate predictions.
Outliers
Outliers (extreme values) in the data can disproportionately affect the regression model, leading to biased or inaccurate predictions.
Multicollinearity
Multicollinearity occurs when two or more independent variables are highly correlated, which can cause the regression model to be unstable and make it difficult to interpret the importance of individual variables.
Lack of Normality
Regression models assume that the residuals (the difference between the observed and predicted values) are typically distributed. If the residuals are not normally distributed, the model may be inaccurate.
Overfitting
Overfitting occurs when a regression model is too complex and captures too much random noise in the data, leading to poor predictions of new data.
Overall, it is essential to carefully evaluate and validate a regression model to ensure that it is accurately capturing the underlying relationship in the data.
Lift Charts
A lift chart is a visual tool that shows the predicted probability of an outcome versus the actual outcome. For example, whether an individual will respond to a marketing campaign. The lift chart is constructed by ranking the predicted probabilities from highest to lowest and then dividing the data into equal-sized bins.
Lift Charts in Statistics
In statistics, lift charts are commonly used to evaluate the performance of predictive models, such as classification or regression models. They provide a visual representation of the relationship between the model's predicted probability of an event occurring and the actual frequency of that event.
This can be useful for identifying how well the model can distinguish between different classes or groups of observations and can help identify areas where the model is performing well or poorly.
To construct a lift chart, the data are first divided into equal-sized bins, such as deciles or quartiles, and the predicted probability and the actual frequency of the event are calculated for each bin. The resulting data are then plotted on a scatter plot, with the predicted probability on the x-axis and the actual frequency on the y-axis.
A line is then drawn from the bottom left to the top right of the plot, called the "lift line," representing a random model's expected performance.
Lift charts are commonly used in marketing and sales to evaluate the effectiveness of predictive models for identifying potential customers or leads.
By comparing the lift line for the model to the lift line for a random model, it is possible to assess how well the model can accurately identify potential customers and areas where the model may be over or under-predicting the likelihood of an event occurring.
Overall, lift charts are a valuable tool for evaluating predictive models' performance and identifying areas where the model may be improved.
Pseudo R-Squared
Pseudo R-squared measures the goodness of fit of a regression model. It is called "pseudo" because it does not have the same statistical properties as the R-squared measure used in traditional linear regression. Pseudo R-squared measures are commonly used in logistic regression, where the outcome is binary (e.g., yes/no, true/false), and the R-squared measure is not applicable.
Several pseudo R-squared measures include the McFadden's R-squared, the Nagelkerke R-squared, and the Cox and Snell R-squared. These measures provide a way to quantify the amount of variance in the outcome explained by the regression model, with higher values indicating a better fit.
However, they should not be compared directly to the R-squared measure from linear regression, as they are not directly equivalent.
Pseudo R-squared measures can help compare the fit of different regression models, but they should not be used as the sole criterion for model selection.
It is essential to consider other factors, such as the interpretability and predictive performance of the model, when choosing the best model for a particular problem.
Market Basket
A market basket is a collection of items typically purchased together by consumers. In retail, a market basket refers to the specific items that a customer adds to their shopping cart during a single transaction.
Market basket analysis is a technique used by retailers to identify products that are commonly purchased together to improve sales and customer satisfaction.
It can be performed using various methods, including association rule mining, cluster analysis, and regression modeling. As a result, it can be a powerful tool for retailers to understand consumer purchasing patterns and drive strategic decisions.
Market Basket Analysis using Logistic Regression
Market basket analysis can employ logistic regression to forecast a customer's propensity to buy a specific good (or set of goods) in light of their previous buying patterns.
To accomplish this, the logistic regression model would be trained using a transaction database that contained records of the goods bought in each transaction.
The things bought in each transaction would serve as the model's independent variables while purchasing a specific item (or collection of items) would serve as the dependent variable.
The logistic regression model would then figure out how the independent and dependent variables relate to one another, using this relationship to forecast the probability that a consumer will buy the target item (or group of things) in the future.
For instance, if "bananas" were the target item, the logistic regression model might forecast that a client who has previously bought milk and eggs is more likely to purchase bananas than a customer who has only bought milk.
By positioning bananas alongside milk and eggs in the supermarket or providing a discount when purchasing bananas along with milk and eggs, this information might then be used to make specific suggestions to customers.
For market basket analysis, logistic regression can be a helpful technique because it offers a quantitative means to comprehend the link between various items and forecast client purchase behavior.
How to Estimate a Market Basket?
Data analysis and machine learning techniques involve several steps in predicting a market basket. The first step is to collect and compile a transaction database containing records of the items purchased in each transaction.
This database can identify the most frequently purchased items (i.e., the market basket) combinations.
Next, a machine learning algorithm can be trained on the transaction database, such as association rule mining or logistic regression.
The goal of the algorithm is to learn the relationship between the items purchased in each transaction and to use this relationship to make predictions about future transactions.
For example, if a customer has previously purchased milk and eggs, the algorithm might predict that they are also likely to purchase bananas in the future.
Once the machine learning model has been trained, it can predict the market basket for new customers. Retailers can use these predictions to make targeted recommendations to customers, such as by placing related items near each other in the store or offering discounts on certain items when they are purchased together.
Overall, predicting a market basket involves the following:
- Collecting and analyzing transaction data.
- Training a machine learning model on this data.
- Using the model to make predictions about future transactions.
This can help retailers improve sales and the shopping experience for customers.
Conclusion
Statistics is a branch of mathematics that deals with data collection, analysis, interpretation, presentation, and organization. It is used in various fields, including science, engineering, finance, and economics, to make informed decisions and predictions based on data.
Some key statistical concepts include probability, random sampling, hypothesis testing, regression analysis, and statistical inference. These techniques help researchers and analysts understand complex data sets and draw conclusions supported by the available evidence.
One of the key advantages of statistics is that it allows researchers to make inferences about a population based on a sample of data rather than collecting data from the entire population.
This makes it possible to study large or complex data sets and draw conclusions that apply to the population.
In addition to its use in research and analysis, statistics is also essential in many other fields, including marketing, finance, and healthcare.
It allows professionals in these fields to make data-driven decisions and better understand their customers, markets, and patients.
Overall, statistics is a valuable field of study with many practical applications and helps researchers and professionals make informed decisions based on data.