Startups Need Analytics Data Stack, Not Just Dashboards
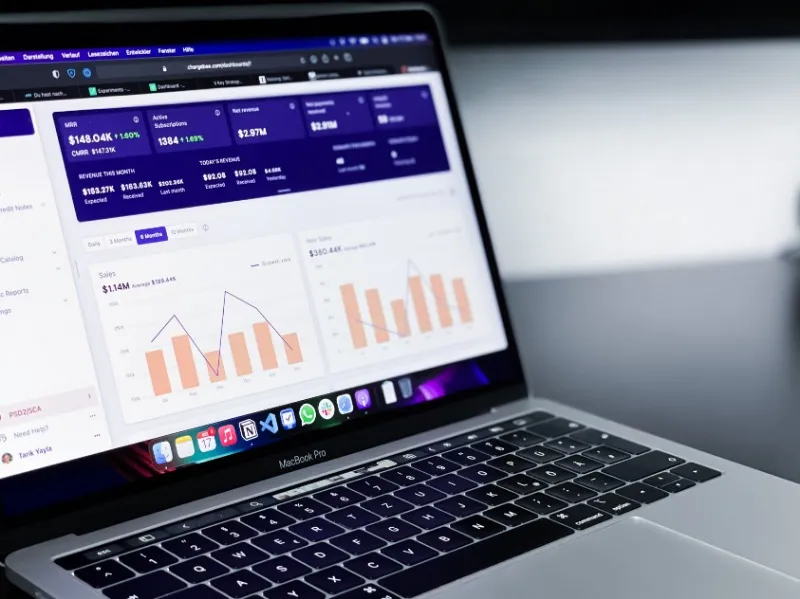
Check out OLake, our latest open source offering for replicating databases to lakehouses.
The world is grappling with how to fully harness the customer data explosion spanning the last few decades. As companies become digital first and marketing stacks get more complex, so does the need for more sophisticated use cases on top of customer data.
Companies across the spectrum still struggle to best apply their data to inform decision-making, build better products, and ultimately delight customers.
Companies at the early stages of their data journey typically deploy a simple architecture focused on data collection and visualization. In this phase, companies collect data from websites and apps.
Integration requests are typically lodged to IT or engineering; the ad-hoc nature of these requests for point-to-point integrations creates a strain on engineering and IT teams.
This is where one-time integrations from products like Datazip and Segment significantly eliminate engineering bottlenecks.
As the company grows, the proliferation of data destinations leads to data silos. Companies will probably hire Data Analysts to make sense of the data across a wide range of business functions.
Out of necessity, the company needs to invest in centralizing data into a warehouse. The warehouse soon evolves into the single source of truth for analytics.
Because analytics data stack is necessary data infrastructure for any company seeking to undertake complex analysis, but not every company has the expertise to navigate infrastructure decisions alone, the need for a solution that is more user friendly became evident. Enter the managed analytics data stack.
Why do startups need an analytics data stack?
Every successful start-up will eventually set up an analytics data stack, but it often happens later than it should. Most will struggle along with managing increasingly complex and large volumes of data in spreadsheets, asking engineering to pull data, and stringing together brittle integrations.
This leads to inconsistent data, biases against making data-informed decisions, and can impact your database performance.
By having an analytics data stack in the early stages of business, companies will avoid these current and future pain points, stay on top of important metrics, get ahead of competitors by having a deeper understanding of their customers, create a data-driven culture from the start, and easily keep investors informed.

How does a modern data stack help early-stage startups?
Marketing attribution across channels
Ad platforms do a great job of telling which ad performs best. But to assess which channel is most effective in producing customers and revenue, startups can't just look at the data that these platforms provide.
This is because they often provide misleading attribution and make it difficult to compare across platforms.
Instead, startups have to combine its ad data with its customer data, and that's really hard to do if they don't have a data stack.
Mitigate customer churn
Data can surface things that are indicators of a customer potentially churning.
Preventing customers from leaving at the moment of churn is a mistake. When customers are indicating they're not getting a lot of value out of the product, that's when they are highly likely to churn.
Startups can only see this happening by combining their product data with their customer data.
Get a deep understanding of customers
When startups have a large number of users or when its users take a rich set of actions, they are no longer able to manage all this information in a spreadsheet or product analytics tool.
This is when they will need to set up a data stack so that they can effectively work with all this data (and exclude non-relevant segments) to not only understand their customers, but also their future behavior.
Build a culture of data from the start
Having a data stack enables non-technical people less reliant on engineering to pull data, so they're able to quickly run experiments, measure results, iterate, and double down on what's working.
Automated reporting for startup and it's current, prospective, and future investors
Reporting on some key metrics doesn't require a data stack. Startups can simply query their database to know key metrics. But having a data stack enables teams to automate this reporting in a BI tool.
Instead of pulling data and juggling spreadsheets every time it is needed to share updates with investors, startups can automate its reports to refresh on a specified cadence and be emailed to individuals.
Not having a separate data stack for analytics slows down analysis, negatively impacts user experience, and makes the transition more painful in the future
- Inconsistencies and multiple answers and uncertainty in whether a goal has been achieved or the progress towards it.
- Querying the production database directly will negatively impact its performance. Website and product will slow down, which harms user experience and can lead to lost sales.
- When startups finally set up an analytics data stack, it'll be painful and time-consuming. Without an analytics data stack, adding more data sources and building more reports will result in clunky ad hoc processes.
The earlier startups put in the right infrastructure, the less challenging it is to make the transition. It's also easier to practice good data practices, such as creating table descriptions and adding data validation, when you only have a small amount of data to manage.
As an early-stage startup, time and resources are precious. It's tempting to put off implementing a analytics data stack, but here's the impact of doing so:
How to set up a modern data stack in an hour
It used to take a few weeks to set up a modern data stack, but there are now options that cut implementation time by 99% and don't require engineering. The barriers to scalable data infrastructure have largely been removed, making it more accessible to early-stage start-ups.
Datazip is an all-in-one, analytics data stack for organizations to ingest, store, transform and visualize data, resulting in fast data-driven decision making, all without the need for expensive data teams. Contact us for more information.