Segmenting Customer Needs and Attitudes
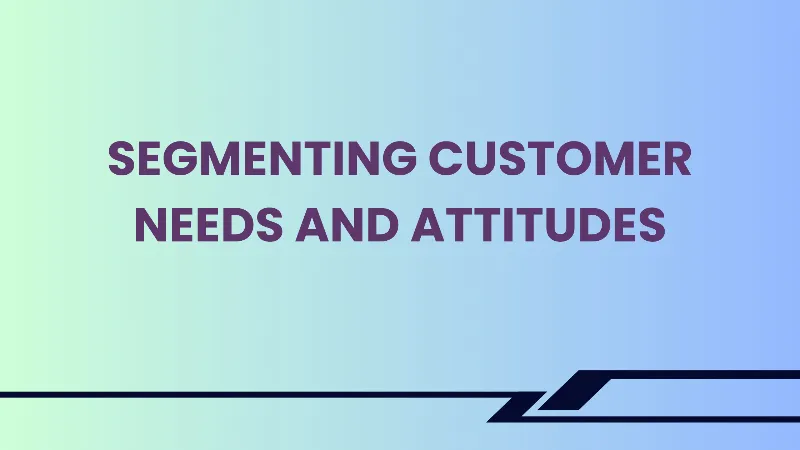
Check out OLake, our latest open source offering for replicating databases to lakehouses.
Do you know what's more important than having a great product or service? It is knowing your customers and what they want! And that's where segmentation and customer analytics come into play.
Segmentation is like sorting your laundry - you don't want to wash your whites with your darks, right? Similarly, you don't want to treat all your customers the same way. By segmenting your customers based on their needs, preferences, and behavior, you can deliver personalized experiences that make them feel special and keep them coming back for more.
Customer analytics is like having a secret weapon - it helps you understand your customers better than they understand themselves! With the power of data analysis, you can uncover valuable insights about your customer's behavior, preferences, and pain points and use that knowledge to make informed decisions about your business strategy.
In this blog, we'll be diving into the wonderful world of segmentation and customer analytics and will show you how to use these tools to supercharge your business.
Segmentation and Customer Analytics
Segmentation and customer analytics are closely related. They both aim to understand customers better. Segmentation involves dividing customers into groups based on shared characteristics, such as demographics, behavior, and interests, while customer analytics uses data analysis techniques to gain insights into customer behavior and preferences.
By combining segmentation with customer analytics, businesses can better understand each group's unique needs and tailor their marketing campaigns and product offerings to improve customer satisfaction and drive growth.
Benefits of Customer Analytics
Customer analytics provides numerous benefits to businesses, including:
- Improved customer insights: By analyzing customer data, organizations may better understand customer behavior and preferences and build more targeted marketing campaigns and products.
- Data-driven decision-making: Customer analytics reduces the risk of costly mistakes in product development and marketing strategy.
- Increased customer satisfaction: By analyzing client behavior and preferences, organizations may offer personalized experiences that boost customer loyalty.
- Improved customer retention: Customer analytics can detect at-risk customers and offer promotions or customized advice to retain them.
- Increased revenue: Tailoring marketing efforts and product offers to client needs can boost sales.
- Competitive advantage: Companies that employ customer analytics to understand and improve customer experience have a competitive edge.
In short, customer analytics is a powerful tool that can help businesses improve their customer insights, make data-driven decisions, increase customer satisfaction and retention, boost revenue, and gain a competitive advantage.
Segmentation Analytics
Segmentation analytics is the process of using data analysis techniques to gain insights into customer segments. It involves analyzing customer data to identify patterns, behaviors, and preferences within each segment.
Factors Affecting Segmentation Analytics
Several factors can affect the success of segmentation analytics, including:

Data Collection in Segmentation
Data for segmentation can be collected through various methods, including:
-
Surveys: Online, phone, and in-person surveys can acquire client data. Structured or unstructured surveys with quantitative and qualitative questions are possible.
-
Customer databases: Customers' buying history, demographics, and contact information can be found in customer databases.
-
Website analytics: Tools can track client behavior on a company's website, including pages visited, duration of visits, and activities.
-
Social media monitoring: Tools for social media monitoring can gather data on customer attitude, engagement, and activity.
-
Focus groups: Customers discuss their experiences and ideas in focus groups. Customer preferences and behavior can be revealed through dialogues.
-
Sales data: Purchase frequency, product preferences, and average order value can be determined from sales data.
The choice of data collection method will depend on the objectives of the segmentation analysis, the data available, and the organization's resources. It is important to ensure the data collected is relevant, accurate, and adheres to privacy regulations.
Customer Segmentation Process
To segment customers effectively, businesses should follow these steps:

Effective customer segmentation can help businesses better understand and target their customers, increasing customer satisfaction, loyalty, and profitability.
Data Analysis for Customer Segmentation
Data analysis is critical to customer segmentation, as it involves identifying patterns and insights that can inform segmentation. Here are the key steps involved in data analysis for customer segmentation:
- Data cleaning and preparation: Before analyzing data, clean and prepare it. This entails deleting irrelevant or duplicate data, repairing errors, and formatting data for analysis.
- Data cleaning and preparation: Before analyzing data, clean and prepare it. This entails deleting irrelevant or duplicate data, repairing errors, and formatting data for analysis.
- Exploratory data analysis: Exploratory data analysis uses descriptive statistics and visualization to acquire insights into the data. This includes identifying data distribution, outliers, and variable relationships.
- Variable selection: Based on exploratory data analysis, choose the most relevant variables for segmentation goals and data.
- Clustering analysis: Clustering clients by similarity is a popular customer segmentation strategy. Customers are segmented using k-means or hierarchical clustering methods.
- Principal component analysis: Data patterns are identified using principal component analysis (PCA). It can identify the key variables that explain data variance and eliminate segmentation variables.
- Regression analysis: Regression analysis predicts customer behavior by identifying variable associations. This can help uncover key customer segmentation factors.
- Evaluation and refinement: After segmentation, segments must be evaluated to verify they are actionable and useful. Adjust segments based on corporate goals and external data.
Overall, practical data analysis is critical for customer segmentation, as it helps businesses to identify patterns and insights that can inform the development of customer segments.
Cluster Analysis
Cluster analysis is a statistical technique that groups object or data points into clusters or segments based on their similarities. The objective is to group objects that are similar to each other and dissimilar to those in other clusters.
Applications of Cluster Analysis
Cluster analysis has a wide range of applications in various fields, including:

Overall, cluster analysis is a powerful tool for identifying patterns and insights in data, and it has a wide range of applications in various fields. For example, businesses and researchers can gain valuable insights that inform their decision-making processes by grouping similar data points or objects into clusters.
Case Studies
Here are a few case studies of customer analysis:
-
Netflix: Netflix recommends movies and TV shows based on consumer data. Netflix can recommend new material based on user viewing history and search queries.
-
Amazon: Amazon customizes product recommendations and promotions based on customer data. Amazon analyzes user data like purchase history and browsing behavior to recommend new products and promotions.
-
Starbucks: Starbucks analyzes customers to personalize marketing and build loyalty. Starbucks may tailor promotions to individual customers by evaluating purchase history and feedback.
-
Coca-Cola: Coca-Cola analyzes its customers to create successful marketing efforts. Coca-Cola can build advertisements that sell by studying customer data like demographics and psychographics.
-
Walmart: Customer analysis optimizes Walmart shop layouts and product offerings. Walmart can determine client preferences by studying purchase history and shopping behavior.
These case studies demonstrate the power of customer analysis in improving business performance and customer satisfaction. Businesses can gain valuable insights to inform their decision-making processes and improve their marketing efforts, product offerings, and customer experiences by analyzing customer data.
Help your customers navigate your website with ease
Data Used for Clustering Analysis
The input data for clustering analysis typically consists of data points, each representing an object, individual, or event being analyzed. Each data point is described by a set of features or variables, which can be either continuous or categorical. The choice of features will depend on the specific application and the goals of the analysis.
Let us understand this topic in depth with Coca-Cola as an example.
Let's say that Coca-Cola wants to segment its customers based on their purchasing behavior. Here are some possible variables or features that could be included in the input data:
- Purchase amount: The amount each customer spends on Coca-Cola products over a specific period.
- Purchase frequency: The number of times each customer purchased Coca-Cola products over a specific time period.
- Product type: The specific Coca-Cola products each customer purchased (e.g., Coke, Diet Coke, Sprite, etc.).
- Purchase location: The location where each customer made their Coca-Cola purchases (e.g., grocery store, gas station, vending machine, etc.).
- Purchase time: The time or day of the week customers made their Coca-Cola purchases.
These variables could be collected from Coca-Cola's point-of-sale systems or customer databases. The input data would consist of a set of customer transactions, where each transaction includes the variables listed above. The clustering algorithm would then be applied to this data to identify groups of customers with similar purchasing behavior.
Finally, the clustering analysis results could be used to develop targeted marketing campaigns or product promotions for each customer segment.
Clustering Algorithms
Clustering algorithms are used to group data points into clusters based on their similarity or distance to each other.
There are generally two classes of clustering algorithms:
Partitioning Clustering
These algorithms partition the dataset into non-overlapping clusters with one data point per cluster. K-Means partitioning clustering is the most popular. K-Medoids, CLARANS, and PAM, are other partitioning techniques.
Hierarchical Clustering
These methods construct a hierarchy of clusters by grouping data points or recursively partitioning the dataset. Two hierarchical clustering algorithms exist:
- Agglomerative clustering: This algorithm merges the two closest clusters at each iteration until all data points are in one cluster.
- Divisive clustering: Divisive clustering (top-down clustering) starts with the complete dataset as one cluster and iteratively breaks it into smaller clusters until each data point is in its own cluster.
Factors Affecting Cluster Formation
There are several factors that can affect the formation of clusters, including:
- Distance metric: Distance metrics affect clusters. Depending on the data, different distance measures may be better.
- Number of clusters: The analyst or search algorithm decides how many clusters to construct. Clusters and analytic interpretation depend on the number of clusters.
- Feature selection: The clusters produced by clustering depend on the features utilized. Feature selection should be based on business challenges and data.
- Scaling: Clustering techniques might be sensitive to feature scale discrepancies. Scaling data ensures that each feature affects clustering analysis equally.
- Outliers: Clustering findings can be affected by outliers. Finding and deleting outliers helps improve clusters.
- Algorithm choice: Clusters can be affected by the clustering algorithm used. Based on data type and business challenge, choose an algorithm.
By carefully considering these factors, analysts can help ensure that the resulting clusters are meaningful and useful for informing decision-making.
How to select the right Clustering Algorithm?
The choice of clustering algorithm and the number of clusters to use can depend on the specific nature of the data and the business problem being addressed. Here are some factors to consider when selecting a clustering algorithm:

It is also important to evaluate the quality of the resulting clusters by examining the cluster profiles, visualizations, and other metrics, such as the silhouette score. Ultimately, the choice of clustering algorithm and number of clusters should be driven by the specific business problem and the nature of the data being analyzed.
Assigning New Customers to Clusters
Assigning new customers to clusters involves using the clustering model developed on historical data to predict the cluster membership of new customers. The process typically involves the following steps:
- Collect data on the new customer: Collect new clients' demographics, buying history, and other pertinent data.
- Preprocess the data: Like historical data, new data points must be preprocessed before clustering.
- Apply the clustering model: Once the data has been preprocessed, the clustering model can be applied to the new data to predict the cluster membership of each new customer.
- Evaluate the results: Check that the anticipated cluster memberships make sense and match the historical data's clusters.
- Take action: New customer marketing and sales can use expected cluster memberships. Marketing messaging and promotions may resonate better with clients in certain clusters.
It is important to note that assigning new customers to clusters may not always be straightforward and may require ongoing refinement of the clustering model as new data becomes available. Additionally, it is important to ensure that the predictions are accurate and meaningful and to avoid overfitting the model to the historical data.
Metrics to track Segmentation
Customer Lifetime Value (CLV)
Definition: It measures the total value a customer brings to a business over their lifetime.
Formula: (Average Purchase Value x Number of Repeat Purchases x Average Customer Lifespan)
Customer Acquisition Cost (CAC)
Definition: It measures the cost of acquiring a new customer.
Formula: (Total Marketing and Sales Expenses / Number of New Customers Acquired)
Customer Churn Rate
Definition: It measures the percentage of customers who stop doing business with a company.
Formula: (Number of Customers Lost / Total Number of Customers)
Net Promoter Score (NPS)
Definition: It measures customer loyalty and satisfaction by asking how likely customers are to recommend a company to others.
Formula: (Percentage of Promoters - Percentage of Detractors)
Customer Segmentation ROI
Definition: It measures the return on investment of marketing campaigns targeted at specific customer segments.
Formula: (Revenue Generated from Segment - Campaign Cost) / Campaign Cost

Conclusion
In conclusion, customer segmentation and analytics are crucial for businesses to effectively target and serve customers. Businesses can identify unique customer segments through data collection and analysis and tailor their marketing and sales strategies to meet their needs and preferences. Clustering algorithms play a significant role in grouping similar customers based on various parameters.
By tracking metrics such as customer lifetime value, acquisition cost, churn rate, net promoter score, and segmentation ROI, businesses can measure the effectiveness of their segmentation and analytics efforts and make informed decisions to improve customer satisfaction and profitability.